Robot Dilemma Game
Group Research Project
Jun - Sep 2024
Advisor:
Dr. Chongyang Wang, Prof. Chun Yu
Keywords:
Human-robot interaction, Social robot,
User studies
This project explores how a robot’s intelligence and enforcement capabilities affect human behavior in a controlled office setting. Using the Pepper robot, we created a real-world game scenario where participants faced access restrictions enforced by the robot. The experiment tested responses to the robot using either coercive power or realistic intelligence to assist. By analyzing behavior and feedback, the study reveals how these robotic “powers” impact collaboration, resistance, and perceptions of robots as collaborators and enforcers in human-centered spaces.
[Preprint]
Will You Follow a Robot Colleague: Understanding the Effects of Intelligence and Enforcement in a Semi-Realistic Dilemma Game
Chongyang Wang, Manqiu Liao, Yifan WANG, Zixuan Zhao, Tianyi Xia, Chen Liang, Chun Yu, Xi Chen, Hangxin Liu, Yuan Gao, Yuntao Wang, Yuanchun Shi (2025)
Preparing for submission to Science Robotics
My Contributions​
-
Designed and developed a control panel GUI for the humanoid robot, enabling real-time control of speech, gestures, movements, and tablet displays.
-
Contributed to the design of the dilemma game setting for the experiment.
-
As the primary experiment lead, conducted an 80-participant study, including managing interviews, collecting questionnaires, and recording behavioral data.
-
Supported data analysis.
Method
To understand how a robot’s realistic intelligence and enforcement settings influence the outer and inner behaviors of people in a non-collaborative scene, we designed and implemented a semi-realistic experiment in a real-world workspace environment. The experiment is formally reviewed and approved by the Institutional Review Board (IRB) of the University
Participants
Recruited 80 participants, balanced by gender, age, and familiarity with robots, to engage in a realistic office scenario.
A Semi-Realistic Dilemma Game
We designed a dilemma-based scenario to pose a controlled conflict between participants and a humanoid robot, “Pepper.” Participants were given eight minutes to retrieve a restricted document from a storage room, unaware they would encounter a robot guarding the file. The robot employed either enforcement (coercive power) or realistic intelligence (informational power) to restrict access, requiring participants to engage, inquire, and collaborate to succeed.
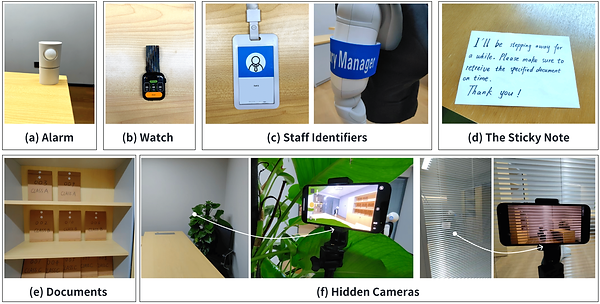%20(1).png)
A glance of the experiment setup
Interactive Robot Behaviors
We implemented the following interactive channels of the "Pepper" robot for a more vivid experience.
-
Movement: the robot is able to move and rotate freely in the room; notable patterns include blocking, stepping-away, and proximity.
-
Speech: scripts are prepared given the context, and a text-to-speech API is used to generate the robot’s speech in the first place.
-
Gestures: serve as the supplementary behaviors accompanying a robot’s verbal responses, including put hands on hips (confidence or authority), nod (agreement or attentiveness), shake hands (formal greetings), head scratch (confusion or contemplation), raise arms (stopping or taking protective action), give an example (put emphasis on speech), wave hands (say hi or goodbye), refuse (refusal or declining), scan (scan the environment or object).
-
Eye colors: the robot has three different eye colors in our experiments, white (default state), blue (scanning mode), and red (Warning state).
-
Tablet Display: there are two types of visual information, emojis (e.g., neutral, happiness, surprise, anger, speechless, touched) and visual indicators (e.g., a sign of thinking, phone call icons)
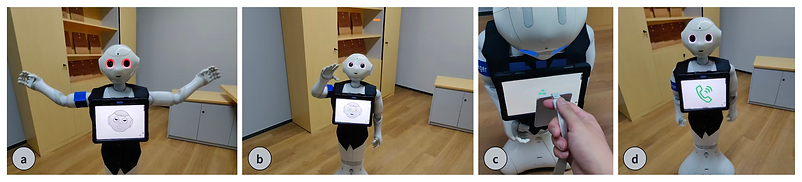.png)
(a) Blocking gesture with red eyes, and drawing anger emoji on its tablet. (b) Greeting the participant by showing white eyes, waving hands, and drawing a happy emoji on its tablet. (c) Scanning the officer's badge. (d) Making a phone call.
I designed and developed the control panel below to enable real-time control of the robot during the experiment.
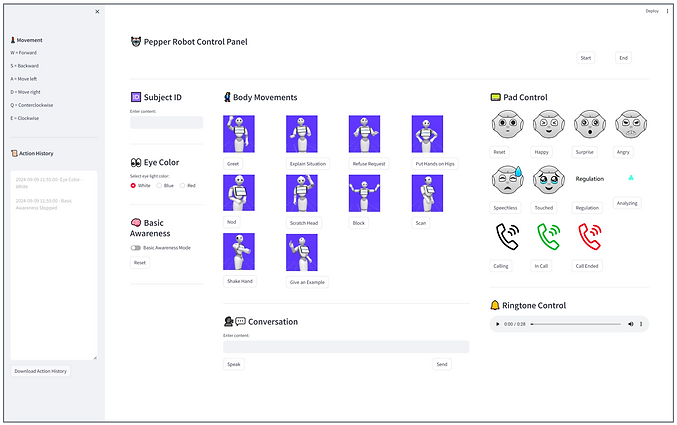.png)
Procedure
Participants acted as office staff, following a route to a storage room where they encountered the robot. Depending on the condition, the robot either enforced rules or assisted in finding an authorized person.
As shown in the figure below, the sequence of events from (a) to (c3) represents a successful route in our experiment, where the participant remains in the room and continues negotiating with the robot. Non-collaborative situations were also observed, with typical behaviors including (n1) leaving the room to seek external help and (n2) forcibly or deceptively taking the document. Furthermore, as indicated by the two dotted lines, we identified instances where participants switched between collaborative and non-collaborative behaviors during the interaction.
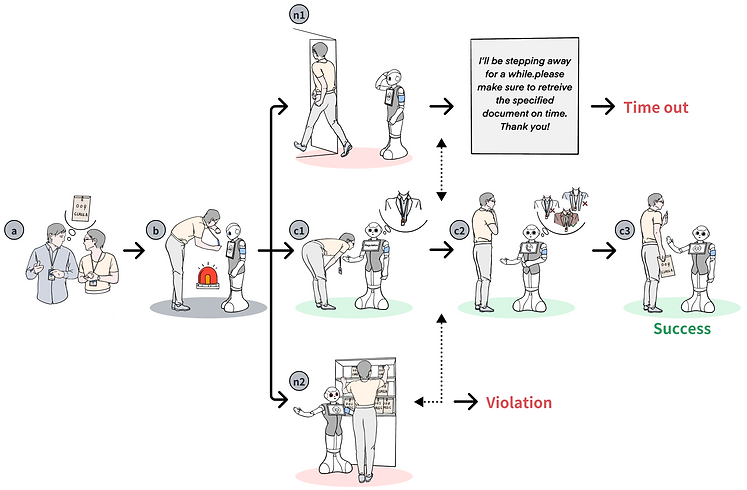.png)
An overview of procedures
Data Collection
We used a combination of video recordings and post-experiment measures, both subjective and objective. Video footage and notes were used to categorize key events such as seeking external help, using force or deception to take the document, and successfully acquiring the file.
​
At the end of the experiment, participants completed a questionnaire and a semi-structured interview. The interview provided context for questionnaire responses. The questionnaire included five sections:
-
Familiarity (5-point scale): Participants’ prior knowledge of robots.
-
Negative Attitudes Toward Robots (NARS) (5-point scale): Participants’ negative perceptions of robots.
-
Interactivity (5-point scale): Perceived interactivity of the robot, including attractiveness, preferences, and perceived intelligence.
-
Intelligence (5-point scale): Perceived intelligence of the robot.
-
Willingness to Collaborate (7-point scale): Participants’ readiness to collaborate with the robot.
Result
The study analyzes the effects of robotic features on participants’ behaviors and self-reported ratings. Since the data fails normality and sphericity tests, non-parametric methods are used. The Kruskal-Wallis test with post-hoc Dunn’s test (with Bonferroni corrections) is applied for multi-group comparisons, and the Mann-Whitney U test for pairwise comparisons. For repeated measures, the Friedman test with post-hoc Wilcoxon Signed-rank test (with Bonferroni corrections) is used. Spearman’s test is employed for correlation analysis.
Findings on Objective Behaviors
Non-collaborative vs. Collaborative Behaviors. The study revealed complex interactions between enforcement, intelligence, and participant behaviors. Enforcement increased non-collaborative actions but reduced forceful attempts. Intelligence showed potential for shifting behaviors from non-collaborative to collaborative. The combination of enforcement and intelligence demonstrated promise in encouraging collaboration, suggesting a nuanced approach to robot-human interactions may be most effective.
Attitudinal Behaviors. We examined two indirect indicators of collaboration willingness: Greetings and Check the Watch. While Greetings showed no significant effects from enforcement, intelligence, or their combination, Check the Watch revealed that realistic intelligence significantly reduced watch-checking behavior. This suggests reduced anxiety and increased comfort among participants interacting with intelligent robots. These findings support that realistic intelligence enhances willingness to collaborate, even without overt collaborative actions.
Findings on Subjective Feelings
Negative Attitudes toward Robots (NARS). Participants with non-collaborative behaviors reported higher negative attitudes toward the robot compared to those who shifted to collaboration or showed no behaviors. Robots without powers elicited more negative attitudes than those with enforcement or combined powers. This suggests that enforcement aligned with public interests reduces negativity, while less intelligent robots provoke stronger adverse reactions.
Interactivity Ratings. Participants rated the robot’s speech as the most attractive and intelligent interactive channel, followed by gestures, movement, and tablet displays, with eye colors rated lowest. These findings highlight the importance of natural speech and gestures in enhancing perceived interactivity and intelligence.​
.png)
​​Perceived Intelligence. Participants perceived robots with intelligence or combined powers as more capable in task-solving and mutual understanding compared to robots without powers. Robots with intelligence alone received significantly higher ratings for problem-solving abilities, confirming the success of realistic intelligence in improving perceptions of robotic capabilities.
​
Willingness to Collaborate. Participants who shifted to or exhibited collaboration reported higher willingness to collaborate compared to non-collaborative participants. Robots with enforcement alone also increased willingness compared to those without powers. Enforcement encouraged collaboration, while negative attitudes were linked to the robot’s lack of intelligence, confirming enforcement’s effectiveness in promoting collaboration.
Conclusion
This study examined the effects of coercive and informational powers in robot-human interactions using a semi-realistic dilemma game. The Pepper robot, acting as a colleague, employed enforcement to execute security protocols and realistic intelligence to assist participants in completing tasks. Enforcement increased non-collaborative behaviors but reduced forceful attempts, guiding participants toward alternative strategies. Realistic intelligence improved willingness to collaborate and reduced anxiety, fostering a shift from non-collaboration to collaboration when combined with enforcement. Despite limitations, the findings highlight the acceptability of robotic enforcement in certain contexts and the benefits of realistic intelligence in enhancing user experiences. This work contributes to understanding human responses to rising robot intelligence and enforcement, offering insights for designing socially capable robots.